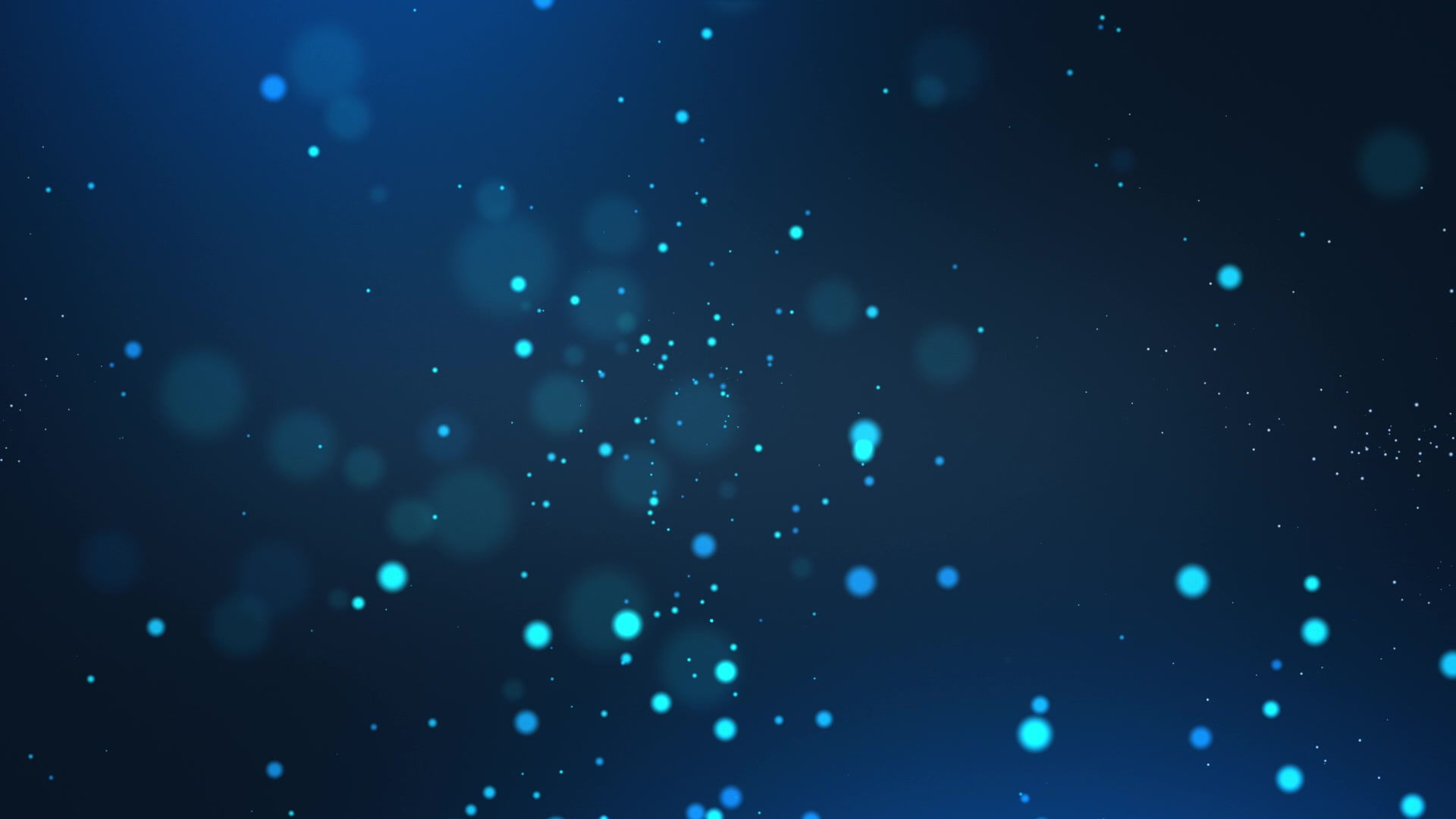
Developing an Artificial Neural Network as a Method for Real-Time Diagnosis and Detection of Atrial Fibrillation in Electrocardiograms
Cell Biology & Biochemistry
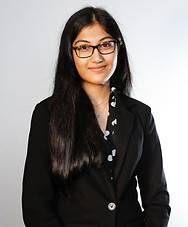
Maryam Imran
is a senior at American Heritage Boca Delray
Maryam Imran is a senior at American Heritage. She is a part of the premedical track and this is her fourth year participating in science research. Last year she was named a Regeneron ISEF finalist and an alum of the Society for Science & the Public.
Abstract
The purpose of this experiment was to develop an artificial neural network in order to accurately diagnose atrial fibrillation. Atrial fibrillation (AF or AFib) is a common type of arrhythmia that results in irregular electrical activity of the heart, in which the atria quiver due to disrupted signal transmission between the SA and AV nodes. The atria are unable to fill the ventricles with blood, and oftentimes blood pools in the heart causing various cardiac complications. Among these are strokes, heart failure, palpitations, pulmonary embolisms, and many others. Atrial fibrillation can be deadly if left untreated, and due to its episodic/asymptomatic nature, it can be difficult to diagnose. Furthermore, diagnosis requires the recording of long electrocardiograms (EKGs), usually hours in duration, and a demanding/tedious search by doctors for AF characteristics in these recordings often yields inaccurate results. This model attempted to accurately diagnose AF with at least 80% accuracy. Data was retrieved from Physionet’s MIT-BIH database. Two different types of patients were used: data from normal sinus rhythm patients and data from AFib patients. The neural network model was trained based on Tensor Flow and Kera’s libraries with Google Colab in Jupyter notebook, while Pandas libraries were used to manipulate data. Results showed a 90% accuracy in diagnosing AFib.